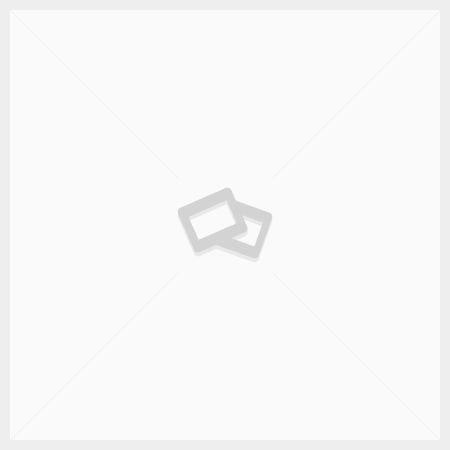
Quantum AI and Neural Networks: Hybrid Models for Enhanced Prediction
In recent years, there has been a growing interest in combining quantum computing and artificial intelligence to create hybrid models that can enhance prediction capabilities. Quantum AI refers to the use of quantum computers in AI applications, while neural networks are a type of machine learning model inspired by the human brain. By combining quantum computing power with the deep learning capabilities of neural networks, researchers hope to achieve significant advancements in predictive modeling.
Quantum computing harnesses the principles of quantum mechanics to perform calculations that are impossible for classical computers. Quantum bits, or qubits, can exist in multiple states simultaneously, allowing quantum computers to explore many possible solutions at the same time. This parallel processing power can significantly speed up complex computations, making quantum computing an attractive option for AI applications.
On the other hand, neural networks are a type of machine learning model that is inspired by the structure and function of the human brain. They are composed of interconnected nodes, or neurons, that process and transmit information. By adjusting the weights of these connections, neural networks can learn from data and make predictions based on patterns and correlations.
When combined, quantum computing and neural networks can create hybrid models that leverage the strengths of both approaches. Quantum AI can speed up the training and inference processes of neural networks, allowing for faster and more accurate predictions. The enhanced computational power of quantum computers can handle larger datasets and more complex models, leading to improved performance across various applications.
One of the key advantages of hybrid quantum AI models is their ability to handle big data and complex problems that are beyond the capabilities of classical computers. Quantum computers excel at solving optimization problems and performing parallel computations, while neural networks can learn from massive datasets and recognize patterns in the data. By combining these two approaches, researchers can create more powerful and efficient predictive models.
Moreover, quantum AI models have the potential to revolutionize industries such as finance, healthcare, and autonomous systems. In finance, quantum AI can be used to optimize investment strategies, predict market trends, and detect fraudulent activities. In healthcare, hybrid models can analyze medical data, identify disease patterns, and personalize treatment plans. In autonomous systems, quantum AI can enhance navigation, decision-making, and real-time adaptation to changing environments.
In order to effectively develop and deploy quantum AI models, researchers and practitioners must overcome several challenges. One of the main challenges is the limited availability of quantum computing resources, as quantum computers are still in the early stages of development and are not yet widely accessible. Additionally, the integration of quantum algorithms with neural networks requires specialized expertise and computational resources.
Despite these challenges, the potential benefits of hybrid quantum AI models are driving significant research efforts in the field. Researchers are exploring new algorithms, hardware architectures, and software tools to improve the performance and scalability of quantum AI models. By advancing the state-of-the-art in quantum computing and artificial intelligence, scientists hope to unlock new opportunities for predictive modeling in the future.
In conclusion, quantum AI and neural networks offer a powerful combination for enhancing prediction capabilities across various applications. By leveraging the strengths of quantum computing and deep learning, researchers can create hybrid models that can handle big data, complex problems, and real-time decision-making. As quantum computing technology continues to advance, the field of quantum AI is poised to make significant strides in predictive modeling and data analysis.
Key quantum ai seriös Takeaways – Quantum AI combines the power of quantum computing with artificial intelligence to enhance prediction capabilities. – Neural networks are machine learning models inspired by the human brain, capable of learning from data and making predictions. – Hybrid quantum AI models leverage the parallel processing power of quantum computers and the deep learning capabilities of neural networks. – Quantum AI has the potential to revolutionize industries such as finance, healthcare, and autonomous systems. – Challenges in developing quantum AI models include limited quantum computing resources and the integration of quantum algorithms with neural networks. – Researchers are actively working to advance the state-of-the-art in quantum computing and artificial intelligence to unlock new opportunities for predictive modeling.